Researchers from the U.S. and China recently published a paper proposing a mindboggling new method of fingerprint theft…
Imagine you get a call from a cybercriminal; or you connect via your smartphone to a conference call that an attacker has access to. During either call, you’d something on your phone, which, naturally enough, involves sliding a finger across its screen. The sound of such a movement is clearly audible through the phone’s built-in mic, allowing the threat actor to record and analyze the sound. From this, they can recreate enough fragments of the fingerprint to unlock your phone using an “artificial finger”! Just think about it: the gentle friction of your finger sliding over the screen can reveal the pattern on the fingertip — a side-channel attack of exquisite beauty!
How to steal a fingerprint through audio
The general schematic of the new PrintListener attack is given in the image:
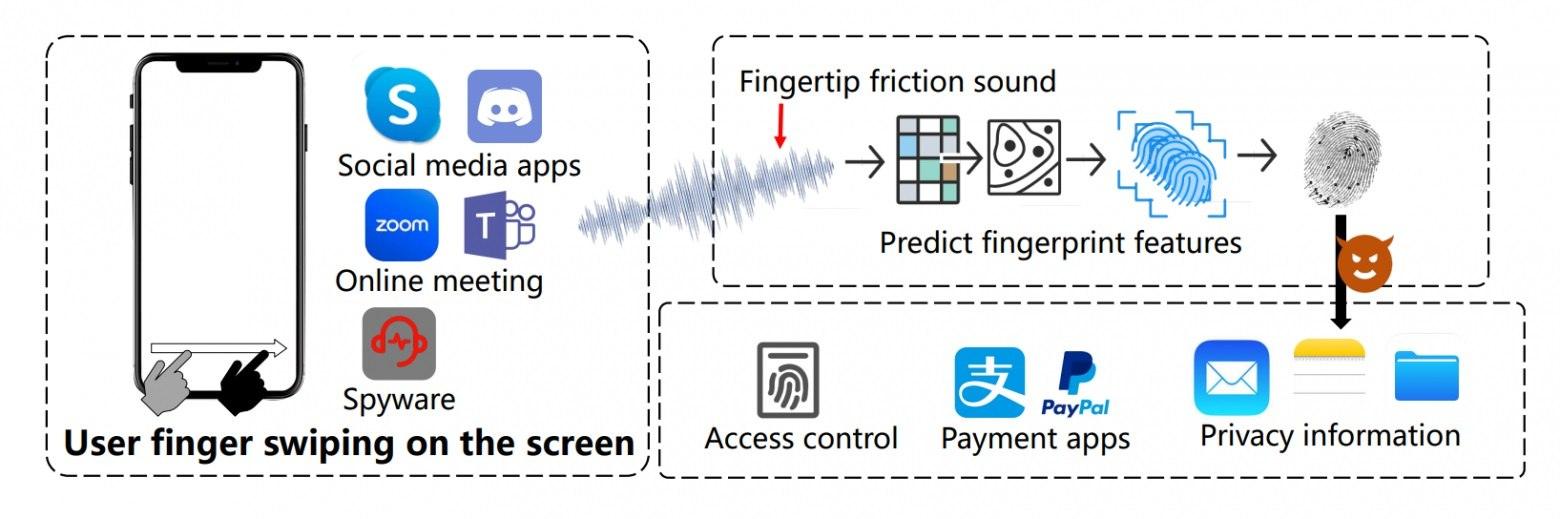
If the potential victim swipes the screen during a call, the attacker can reconstruct parts of the fingerprint from the sound it makes. Source
When the user moves a finger across the surface of the screen, it produces a noise almost inaudible to the human ear. These “rustling” sounds differ depending on which particular loops, arches, and swirls and whirls on the fingertip come into contact with the screen. If the noise is captured by the device’s mic and later analyzed, based on the data obtained, the approximate pattern of these ridges can be determined.
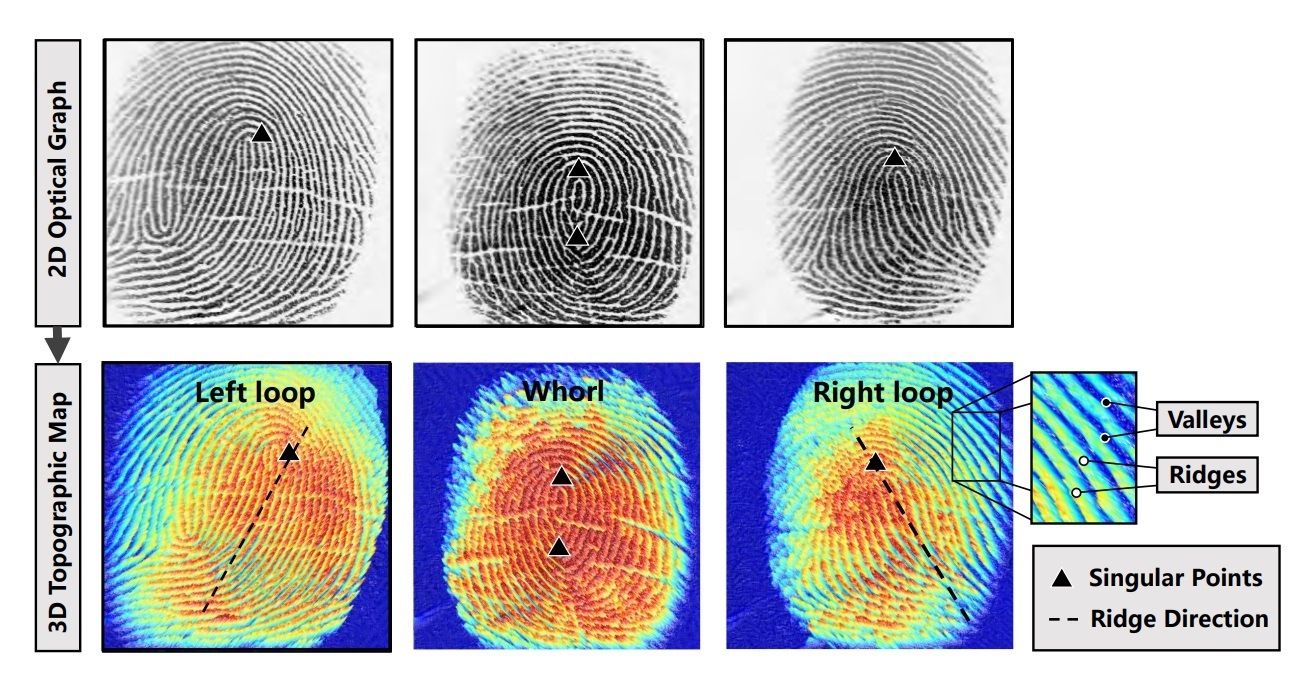
The pronounced features of a fingerprint are key to determining whether a scanned print is identical to the one previously saved. Source
The authors of the paper took great pains to make the study as true to life as possible. First, to avoid having to find such hard-to-detect events manually, they created an automated system to search for sounds similar to a finger being swiped across the screen. Second, they created a large database of photos of fingerprints and the corresponding sounds of finger swipes in different directions, with different background noise, for different smartphone models, and other parameters.
A total of 65 volunteers took part in the experiment, in which 180 fingers were scanned. The data was processed by a machine-learning algorithm. The trained algorithm was able to predict with confidence certain fingerprint characteristics solely by the sound of finger movement across the surface of the smartphone.
How effective is PrintListener?
PrintListener is by no means the first attack on fingerprint scanners. In 2017, a paper was published laying out a scheme in which, instead of the user’s real fingerprint, a synthetic one with random fingerprint patterns was applied to the scanner. And in some cases, it worked! Why? In many modern smartphones, the fingerprint scanner is built into the power button and is pretty narrow. By definition, such a scanner can only see a fragment of the fingerprint. What’s more, the scanner is focused squarely on the pronounced features of the fingerprint pattern. If some loop or swirl on the synthetic finger matches any on the real one, the scanner can authorize the user! The attack was dubbed MasterPrint.
Another important parameter of scanner performance is the rate of false positives. The ideal scanner should only validate a fingerprint if the pattern is a 100% match. But such perfection is unworkable in the real world. Two swipes are never the same — the user’s finger may be at a different angle, a little higher, or a little lower. The finger may be dry or wet, dirty or cut. To take this into account, the scanner is configured to validate not only 100% matches but “good enough” ones as well. This inevitably leads to false positives: when the scanner mistakes a wrong print for the true one. The typical percentage of unwanted positives varies from 0.01% (in the strictest case) to 1%. The latter makes life easier for the user but increases the likelihood that someone else’s finger could unlock the device.
The MasterPrint attack showed that a synthetic fingerprint with some similarly shaped loops or swirls was partially recognized in 2.4–3.7% of cases — and on the first try at that. If multiple attempts are allowed, the likelihood of a false positive rises considerably. In the study, given 12 consecutive swipes, a fake fingerprint got validated 26–30% of the time! In those experiments, the false positive rate was 0.1%.
The PrintListener attack takes the ideas of the 2017 MasterPrint paper and develops them further. Processing the audio information permits detection of the presence of pronounced ridges with a high degree of certainty. This then makes it possible to attack the scanner not at random, but using a fingerprint feature reconstructed from the audio. An attacker can then 3D-print a finger with a synthetic fingerprint that contains this feature.
With an acceptable false positive rate of 0.1%, the PrintListener attack successfully duped the fingerprint scanner 48–53% of the time. A more stringent scenario, with an acceptable false positive rate of 0.01%, still saw the biometric scanner get hacked in 7.8–9.8% of cases. That’s a significant improvement on MasterPrint. Moreover, in each case, no more than five attempts were made to scan the synthetic finger, which corresponds to real-life restrictions on biometric authorization in these same smartphones.
Biometrics pros and cons
We covered the traditional risks associated with fingerprint scanners in a previous post. In short, they’re not an ideal means of authorization in any way. It’s actually quite easy to steal your fingerprint using traditional methods. People always leave fingerprints on the objects and surfaces they touch. In some cases, it’s even possible to extract a usable print from a photograph. And not just from a close-up of your fingers — an ordinary high-res shot taken from a reasonable distance of three meters would do.
The simplest scanners can be fooled by a printout of stolen biometric information. This trick won’t work with the ultrasonic sensors found under modern smartphone displays, but, again, it’s possible to 3D-print an artificial finger with the required pattern. A problem common to all biometric authentication systems is that such information is hard to keep secret. And, unlike a password, you can’t change your fingerprint if it’s compromised.
That’s not to say that the new paper gives new reasons to worry about our data security. The imperfect nature of biometrics is already factored into the logic of the sensors in the devices we use. It’s precisely because a fingerprint is fairly easy to misrecognize that smartphones regularly ask us to enter a PIN or confirm an online purchase with a password. In combination with other security methods, fingerprint scanners aren’t all that bad. Such protection against unauthorized access is better than none at all, of course. Remember, too, that a simple digital unlock code for a smartphone can also be snooped or brute-forced based on traces left on the display.
Nevertheless, the PrintListener attack is indeed remarkable, allowing as it does to pull valuable fingerprint data from the unlikeliest of sources. The attack scenario also looks quite realistic —similar in concept to previous studies in which user keystrokes were recognized by sound. One might conclude from all this that it’s best to refrain from touching your screen during a call or online meeting. But the moral of the story is actually simpler: don’t protect highly sensitive information — especially confidential business-related data — with biometrics alone.